
GraphWatch: Semi-automated threat hunting with graph neural networks for automation environments
In the context of Industry 4.0 and advancing digitalization, the networking of operational technology (OT) to cyber-physical systems (CPS) is becoming increasingly comprehensive. This creates multiple interfaces that offer an increased attack surface for cyber attacks. Protection against these attacks is becoming increasingly important due to the growing global threat situation, especially in the context of critical infrastructures. While simple attacks can be prevented using established methods (if they are used at all), advanced persistent threats (APTs) pose a particular danger due to their sophisticated capabilities and domain knowledge. The complexity of production environments, the heterogeneity of components and the difficulty of using security measures established in the IT world pose particular challenges for the detection of attacks. The often weaker perimeter protection and a large number of remote maintenance access points make compromise more likely and therefore require a strong ability to detect attack activities within a network. This is exacerbated by the fact that production environments are becoming increasingly flexible and modular.
In this research project, methods are to be developed that are specifically designed to detect APT attacks and the techniques and tactics used in CPS. The chosen approach is designed as threat hunting, complementary to classic security monitoring/SIEM (Security Information and Event Management). Threat hunting is an approach to detecting cyber threats in which security analysts specifically search for anomalies in IT systems in order to identify unknown or advanced threats before they can cause damage. In this project, threat hunting is to be semi-automated, with anomaly detection in the network automatically generating indications of anomalous behavior and manual threat hunting taking place in the next step. Graph-based methods are the state of the art for APT attack detection. The aim of the research project is to eliminate the weaknesses of these existing methods in APT attack detection and to enable universal long-term operation in real system environments that can also cope with changing system states.
As part of the project, inIT is working together with other project partners. Hannover University of Applied Sciences and Arts (HsH) has extensive expertise in various areas of anomaly detection for the purpose of attack detection and will develop a detection system in this project. For this purpose, a graph representation is planned that can work with different system types and levels of detail of the data to be mapped. Based on the concepts of the HsH, rt-solutions.de GmbH is in charge of developing a demonstrator that will be evaluated in terms of its suitability for practical use. inIT will develop a methodology for modeling digital twins, which will map security-relevant information for CPS and be made available to the general public as a universal test landscape for threat hunting in the form of open source software. To this end, inIT is contributing its expertise and the SmartFactoryOWL as a realistic field of application.
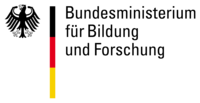
