
IMPROVE: Innovative Modeling Approaches for Production Systems to Raise Validatable Efficiency
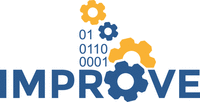
Within the manufacturing industry, the complexity of production plants is steadily rising due to increasing; product variances, product complexity, and pressures for production efficiency. Production systems must therefore now evolve rapidly and operate optimally, creating challenges for larger Industries and serious problems for SMEs without the needed expertise or sufficient resources to adapt new technical possibilities.
The IMPROVE research and innovation project aims to develop Human Machine Interface (HMI) solutions that can be standardised, commercialised, made accessible and applicable for European SMEs by tackling the problem of user support functions in terms of self-diagnosis and self-optimisation.
Alternatively to relying on human expertise and engineering to formulate necessary knowledge, self-diagnosis and optimisation of production plants will be performed in a data-driven way utilizing recent innovations in ICT that support synchronised recording and integration of all production plant sensor values.
Applying concepts from the field of big data, this input will be analysed thoroughly, developing model learning algorithms that describe system behaviour. This greatly extends capacities of manual creation, making it possible to learn accurate virtual factory models of complex, large, and distributed plants through use of real time analytics.